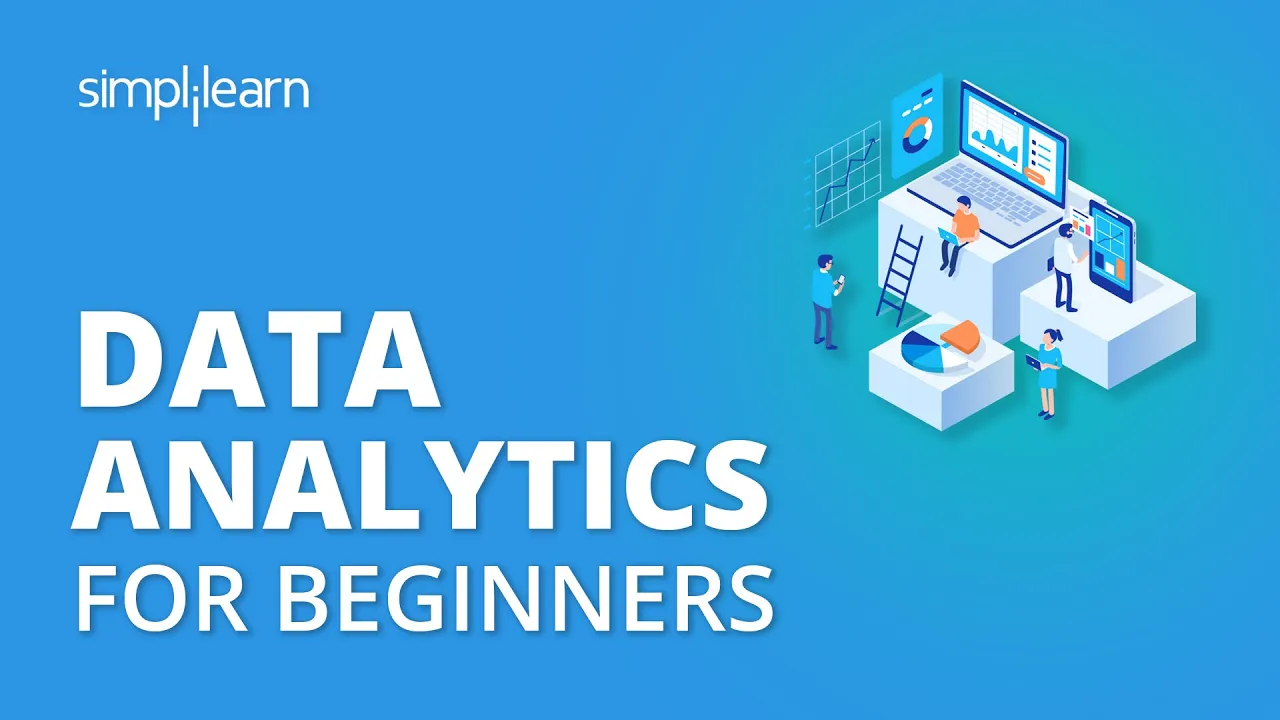
Data Analytics For Beginners | Introduction To Da…
July 18, 2023
Ka siwaju
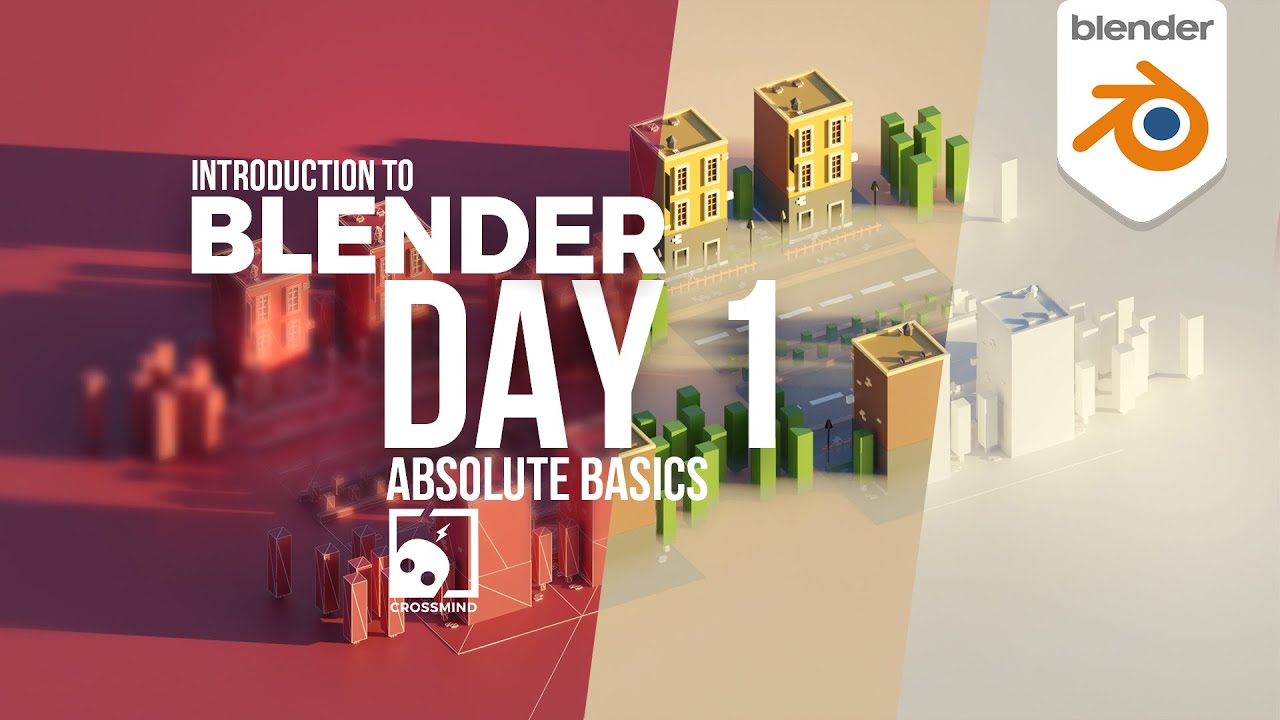
Blender Day 1 - Absolute Basics - Introduction Se…
July 25, 2023
Ka siwaju
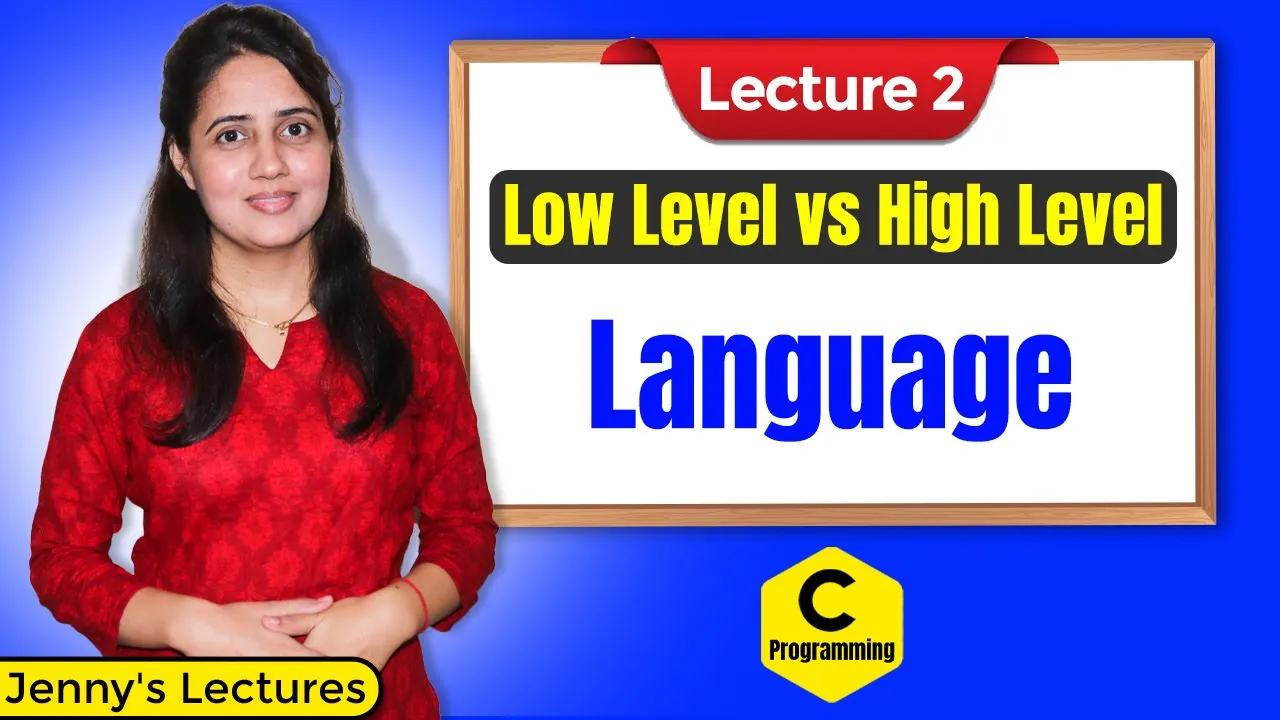
C_02 Low level vs High level Languages | Machine …
July 22, 2023
Ka siwaju

Introduction to ScienceLogic SL1: A Demo with Nic…
July 13, 2023
Ka siwaju
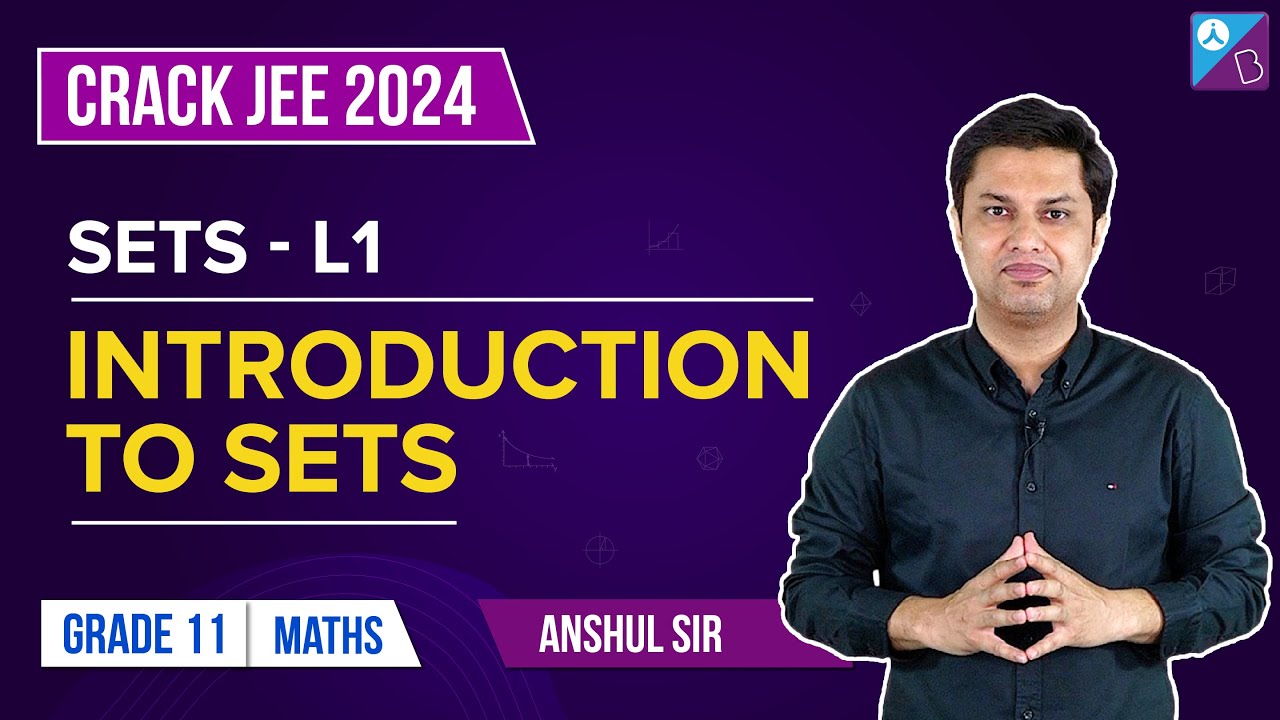
Introduction to Sets & Venn Diagram | Class 11 Ma…
July 19, 2023
Ka siwaju
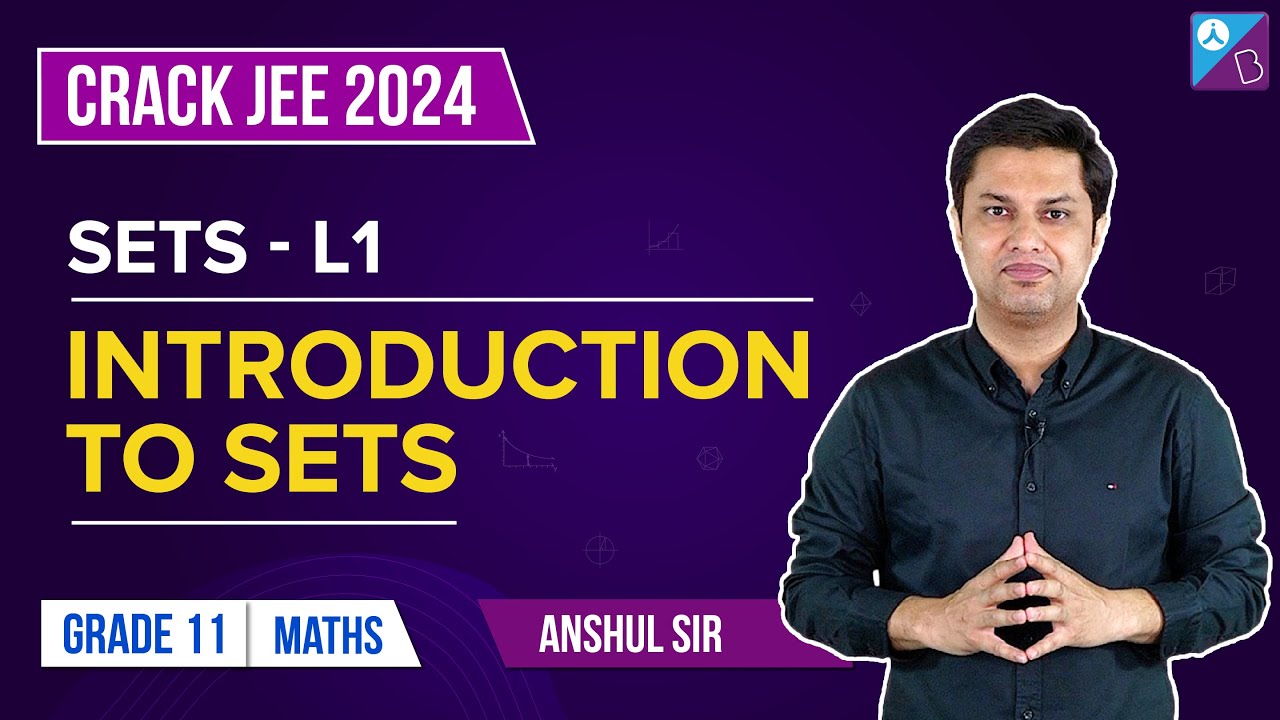
Introduction to Sets & Venn Diagram | Class 11 Ma…
July 19, 2023
Ka siwaju
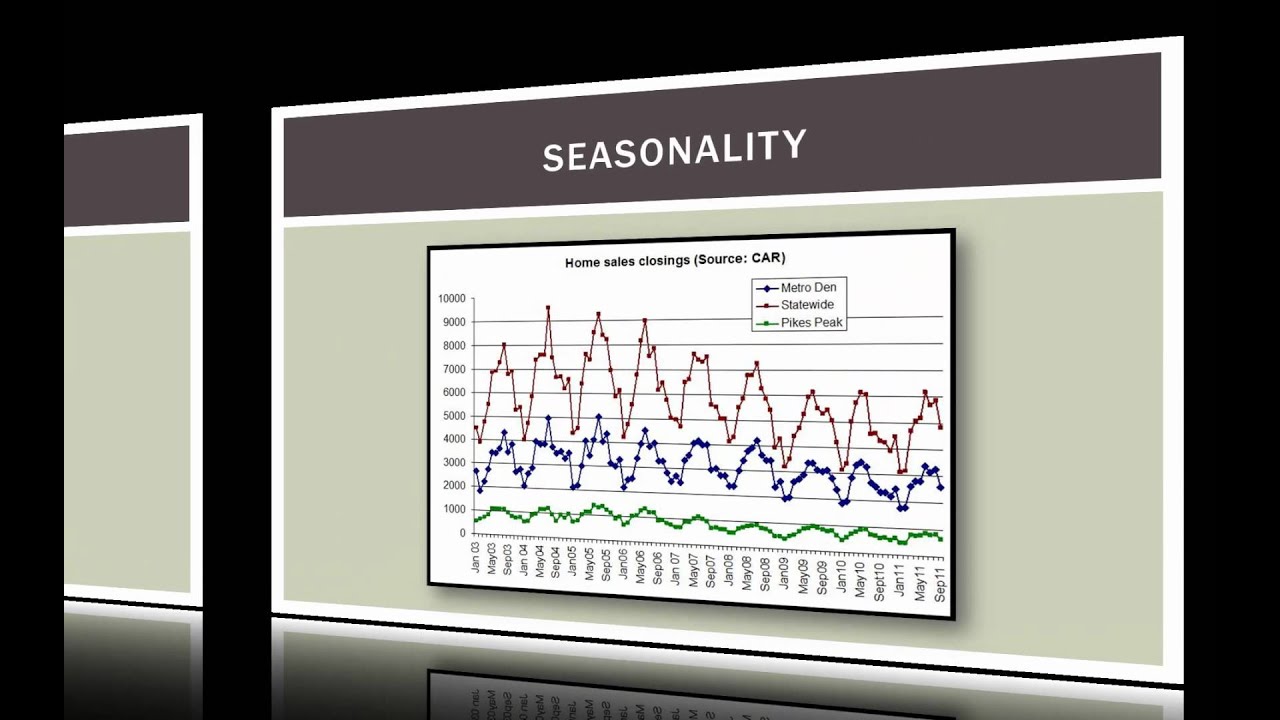
Operations Management 101: An Introduction to Tim…
Aug. 4, 2023
Ka siwaju
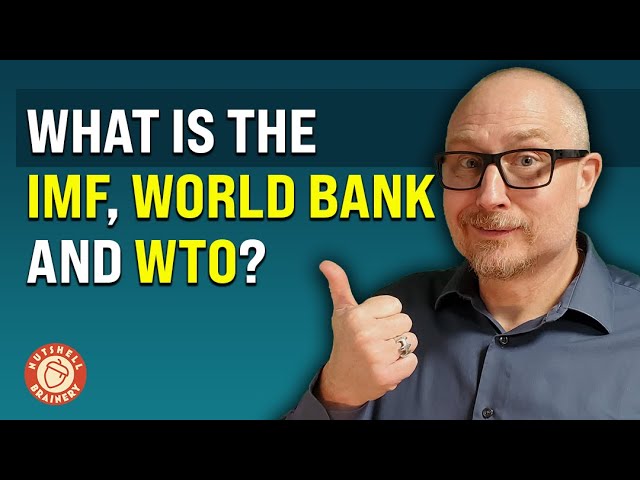
What Is the IMP, World Bank, and WTO? - Module 12
Aug. 11, 2023
Ka siwaju
Introduction to Digital Marketing - What is Digit…
July 25, 2023
Ka siwaju
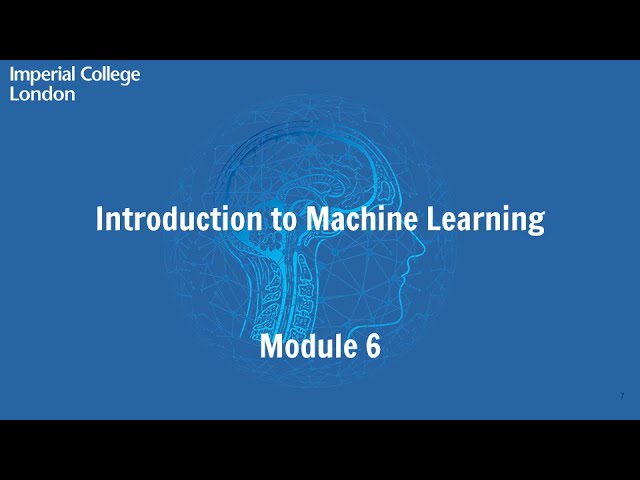
Introduction to Machine Learning: Module 6.9 GMM-…
March 11, 2024
Ka siwaju
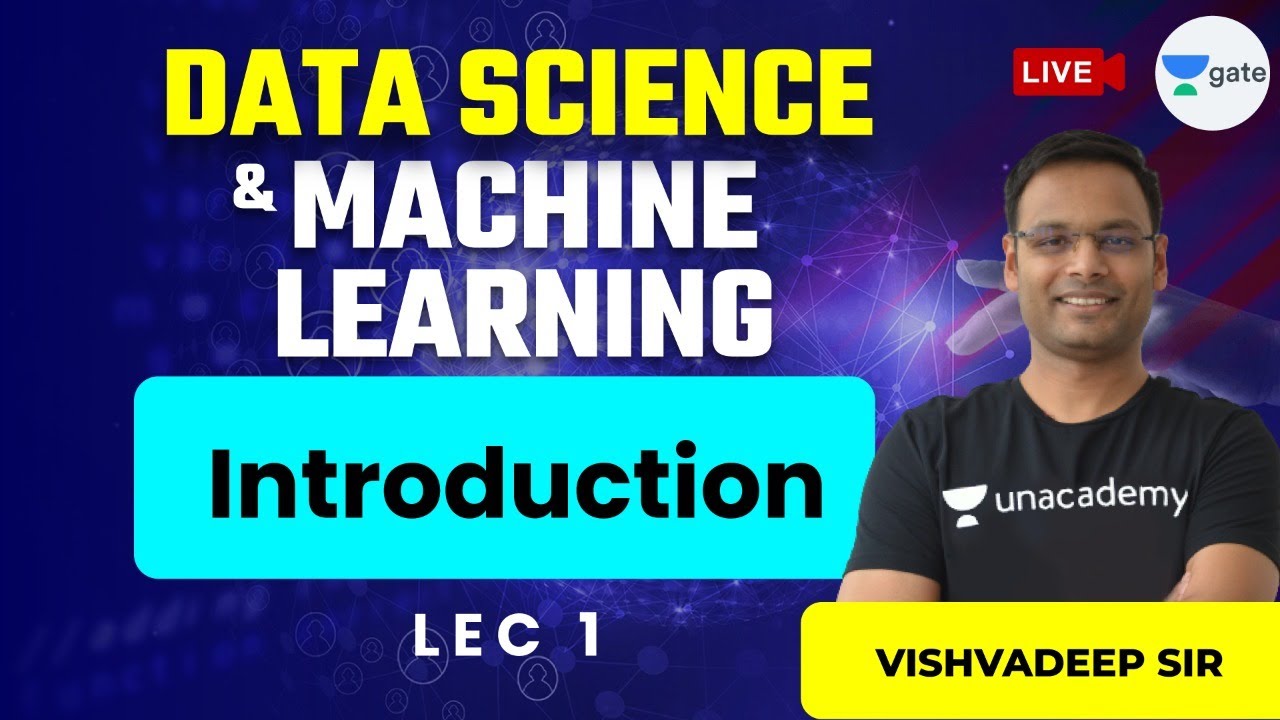
Introduction to Data Science | L - 1 | Data Scien…
July 14, 2023
Ka siwaju
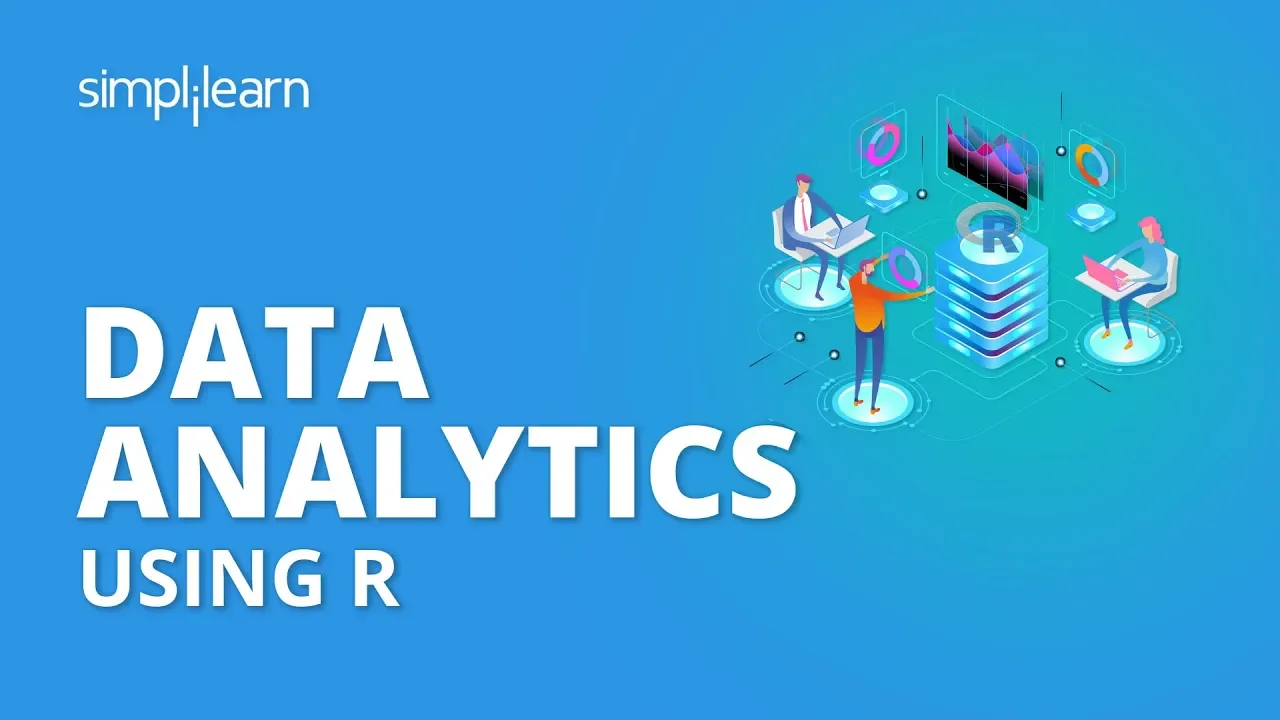
Data Analytics Using R | Introduction To Data Ana…
July 19, 2023
Ka siwaju
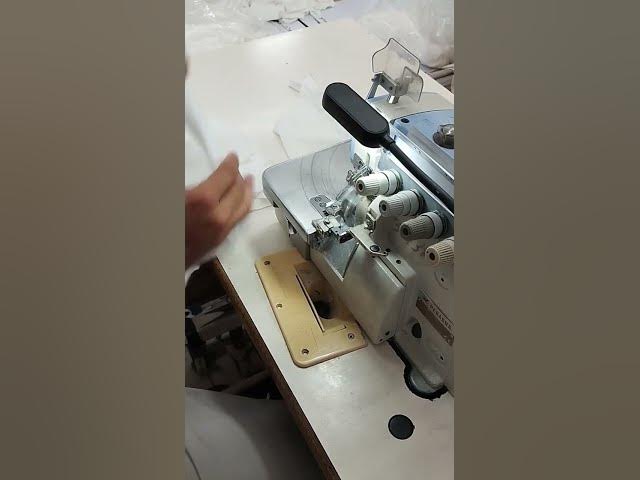
Cuff Blind Hem #handskills #stitching #machine #v…
May 12, 2024
Ka siwaju